本期为大家推荐的内容为论文《Measuring Physical Disorder in Urban Street Spaces: A Large-Scale Analysis Using Street View Images and Deep Learning》(城市街道空间物理无序的测度:基于街景图像和深度学习的大规模分析),发表在Annals of the American Association of Geographers 期刊,欢迎大家学习与交流。
城市空间失序与经济状况、公共健康和社会稳定的负面结果相关, 如财产贬值、精神压力、恐惧和犯罪。目前, 对城市空间失序、尤其是在精细尺度上识别城市空间失序的研究较少但有增多的趋势。然而, 现在还没有一种有效、可复制和低成本的方法来评估大范围的城市空间失序。为了填补这一空白, 本文以海量街景图像作为虚拟核查的输入数据, 利用深度学习模型定量评估城市街道空间失序。我们在中国70多万条城市街道上实现了这一方法, 结果证明了该方法的有效性和效率。据我们所知, 这是全球首次尝试量化如此大规模城市区域的空间失序。通过在中国的大规模实证分析, 本文在理论上有如下几点贡献。首先, 我们拓展了以前对美国的研究中被忽视的城市空间失序因素。其次, 我们发现, 城市空间失序呈现出三种典型的空间分布:分散型、扩散型和线性集中型, 这为揭示城市空间失序的发展趋势和空间干预提供了参考。最后, 对城市空间失序和街道特征之间的回归分析, 确定了城市空间失序的可能影响因素, 从而丰富了理论基础。
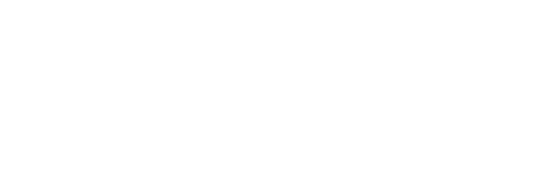
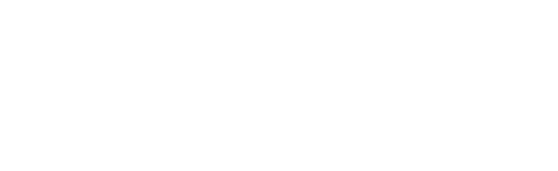
题目:Measuring Physical Disorder in Urban Street Spaces: A Large-Scale Analysis Using Street View Images and Deep Learning
(城市街道空间物理无序的测度:基于街景图像和深度学习的大规模分析)
作者:Jingjia Chen, Long Chen, Yan Li, Wenjia Zhang & Ying Long
发表刊物:
Annals of the American Association of Geographers
DOI:
https://doi.org/10.1080/24694452.2022.2114417
摘要ABSTRACT
Physical disorder is associated with negative outcomes in economic performance, public health, and social stability, such as the depreciation of property, mental stress, fear, and crime. A limited but growing body of literature considers physical disorder in urban space, especially the topic of identifying physical disorder at a fine scale. There is currently no effective and replicable way of measuring physical disorder at a fine scale for a large area with low cost, however. To fill the gap, this article proposes an approach that takes advantage of the massive volume of street view images as input data for virtual audits and uses a deep learning model to quantitatively measure the physical disorder of urban street spaces. The results of implementing this approach with more than 700,000 streets in Chinese cities—which, to our knowledge, is the first attempt globally to quantify the physical disorder in such large urban areas—validate the effectiveness and efficiency of the approach. Through this large-scale empirical analysis in China, this article makes several theoretical contributions. First, we expand the factors of physical disorder, which were previously neglected in U.S. studies. Second, we find that urban physical disorder presents three typical spatial distributions—scattered, diffused, and linear concentrated patterns—which provide references for revealing the development trends of physical disorder and making spatial interventions. Finally, our regression analysis between physical disorder and street characteristics identified the factors that could affect physical disorder and thus enriched the theoretical underpinnings.
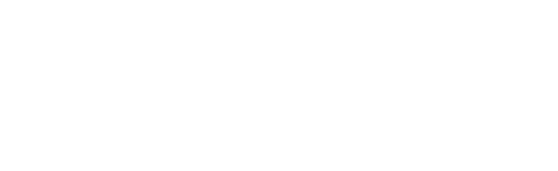
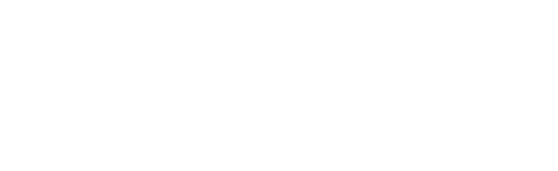
更多内容,请点击微信下方菜单即可查询。
请搜索微信号“Beijingcitylab”关注。
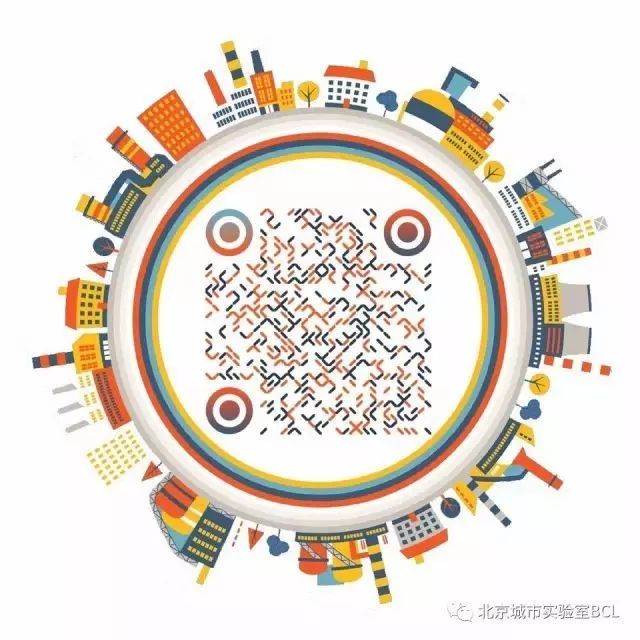
Email:BeijingCityLab@gmail.com
Emaillist: BCL@freelist.org
新浪微博:北京城市实验室BCL
微信号:beijingcitylab
网址: http://www.beijingcitylab.com
责任编辑:张业成、孟庆祥
原文始发于微信公众号(北京城市实验室BCL):论文推荐 | 城市街道空间物理无序的测度:基于街景图像和深度学习的大规模分析