本期为大家推荐的内容为论文《Understanding Bikeability: Insight into the Cycling-City Relationship Using Massive Dockless Bike-Sharing Records in Beijing》(理解城市可骑行性:利用北京大规模无桩共享单车数据洞察城市与骑行的关系),发表在International Conference on Computers in Urban Planning and Urban Management会议,收录在CUPUM 2023: Intelligence for Future Cities上,欢迎大家学习与交流。
来自新兴的无桩共享单车的骑行数据为深入了解多种精细化的骑行特征与建成环境要素之间的关系提供了新的机会。本研究以北京市为例,以街道为分析单元,在控制其他建成环境要素基础上,检验了三种骑行特征与空间视觉元素之间的关联。结果显示,大多数视觉元素与骑行特征显著相关,但它们在骑行距离、速度和流量模型中的表现不同。结果还表明,长距离或快速骑行的个体更喜欢有更多天空和绿色景观视野的街道。较宽的且空间失序较少的街道往往有更高的骑行量。这些发现可以增强对骑行行为的理解,并促进更适宜骑行的城市街道设计实践。
题目:Understanding Bikeability: Insight into the Cycling-City Relationship Using Massive Dockless Bike-Sharing Records in Beijing
(理解城市可骑行性:利用北京大规模无桩共享单车数据洞察城市与骑行的关系)
作者:Enjia Zhang, Wanting Hsu, Ying Long*, and Scott Hawken
发表会议:
International Conference on Computers in Urban Planning and Urban Management
DOI:
https://doi.org/10.1007/978-3-031-31746-0_7
URL:
https://link.springer.com/book/10.1007/978-3-031-31746-0
Cycling records from emerging dockless bike-sharing services provide new opportunities to gain insight into the interactions between multiple fine-scale cycling characteristics and built environmental elements. Using Beijing as an example and the street as the analytic unit, this study examined the associations between three cycling characteristics and spatial visual elements while controlling for other built environmental features. The results showed that most visual elements were significantly associated with cycling characteristics, but their performance differs across models for trip distance, speed, and volume. The results also indicated that individuals riding long distances or at fast speeds preferred streets with more sky and greenery views. Likewise, wider streets with less spatial disorder, tended to have a higher riding volume. The findings can enhance the understanding of cycling behaviors and promote the implementation of urban design for more bikeable streets.
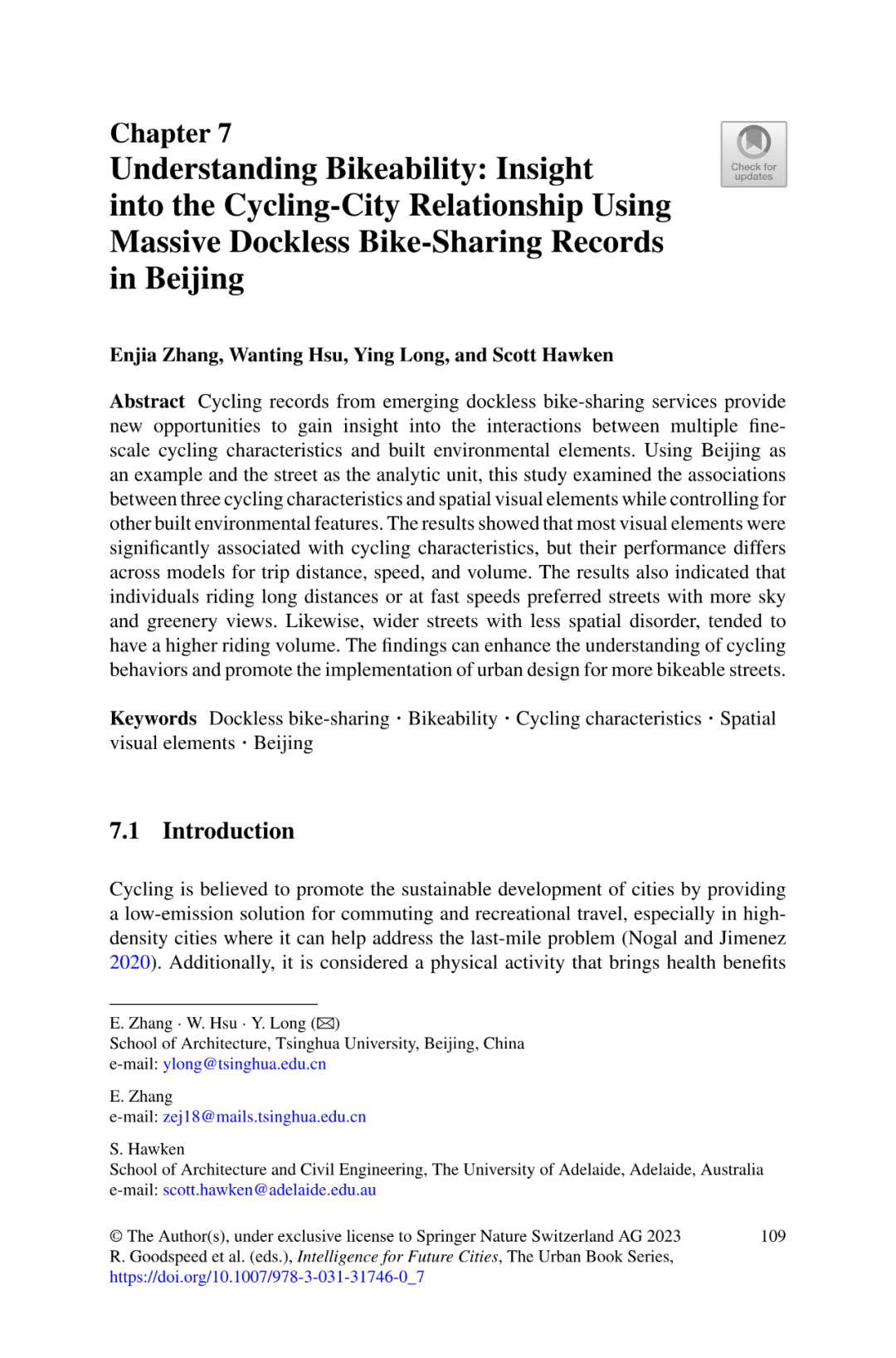
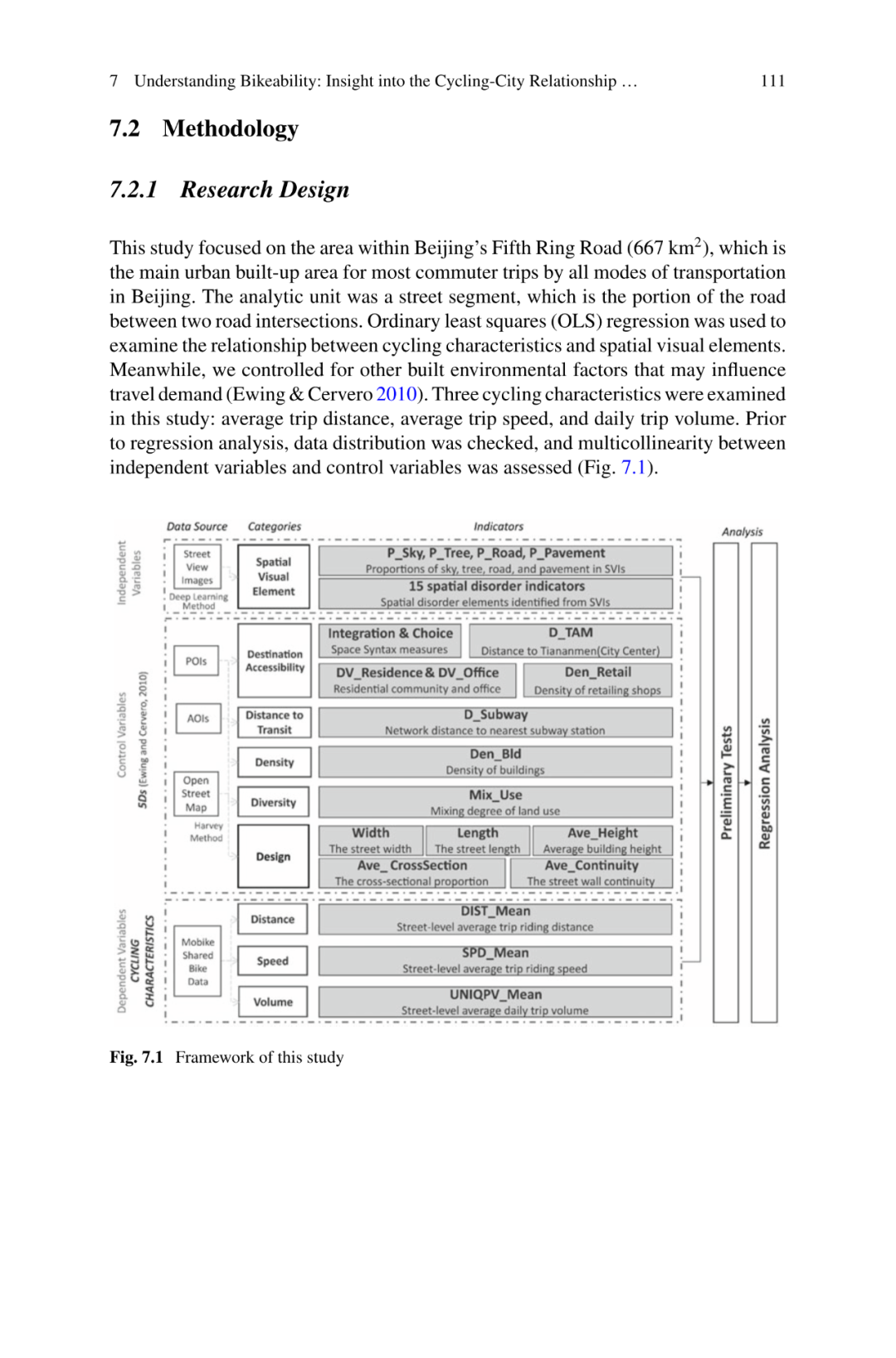
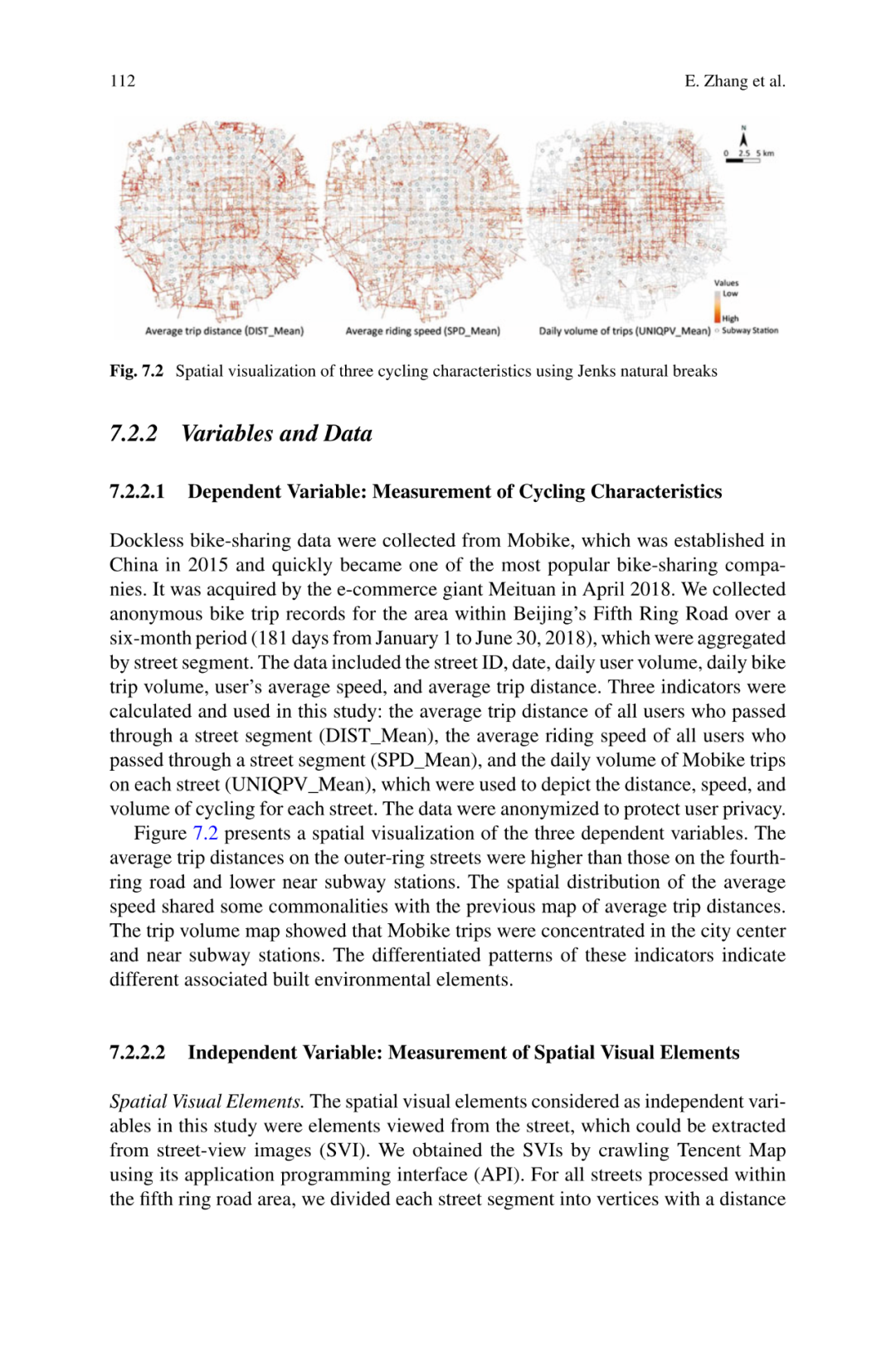
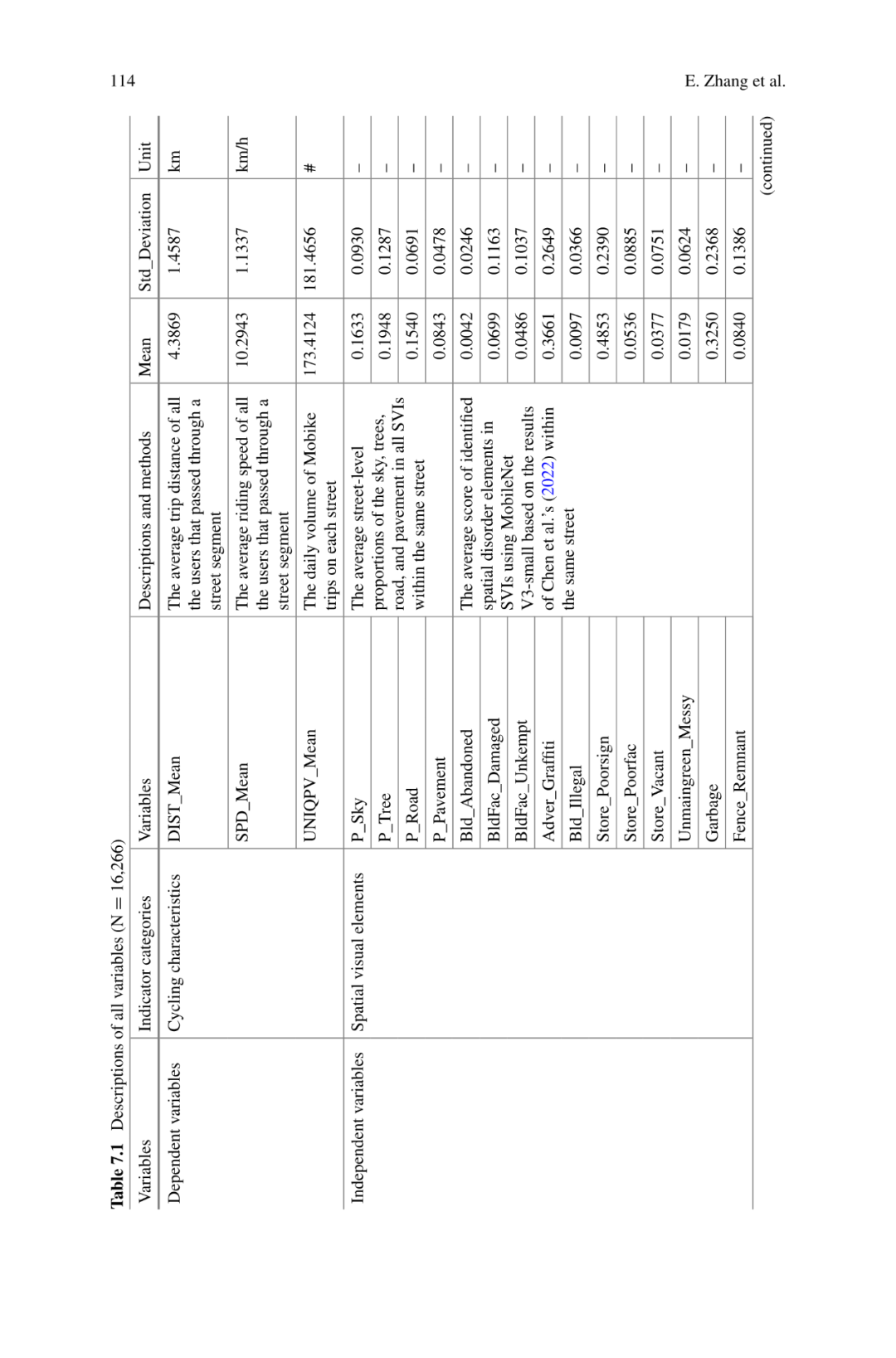
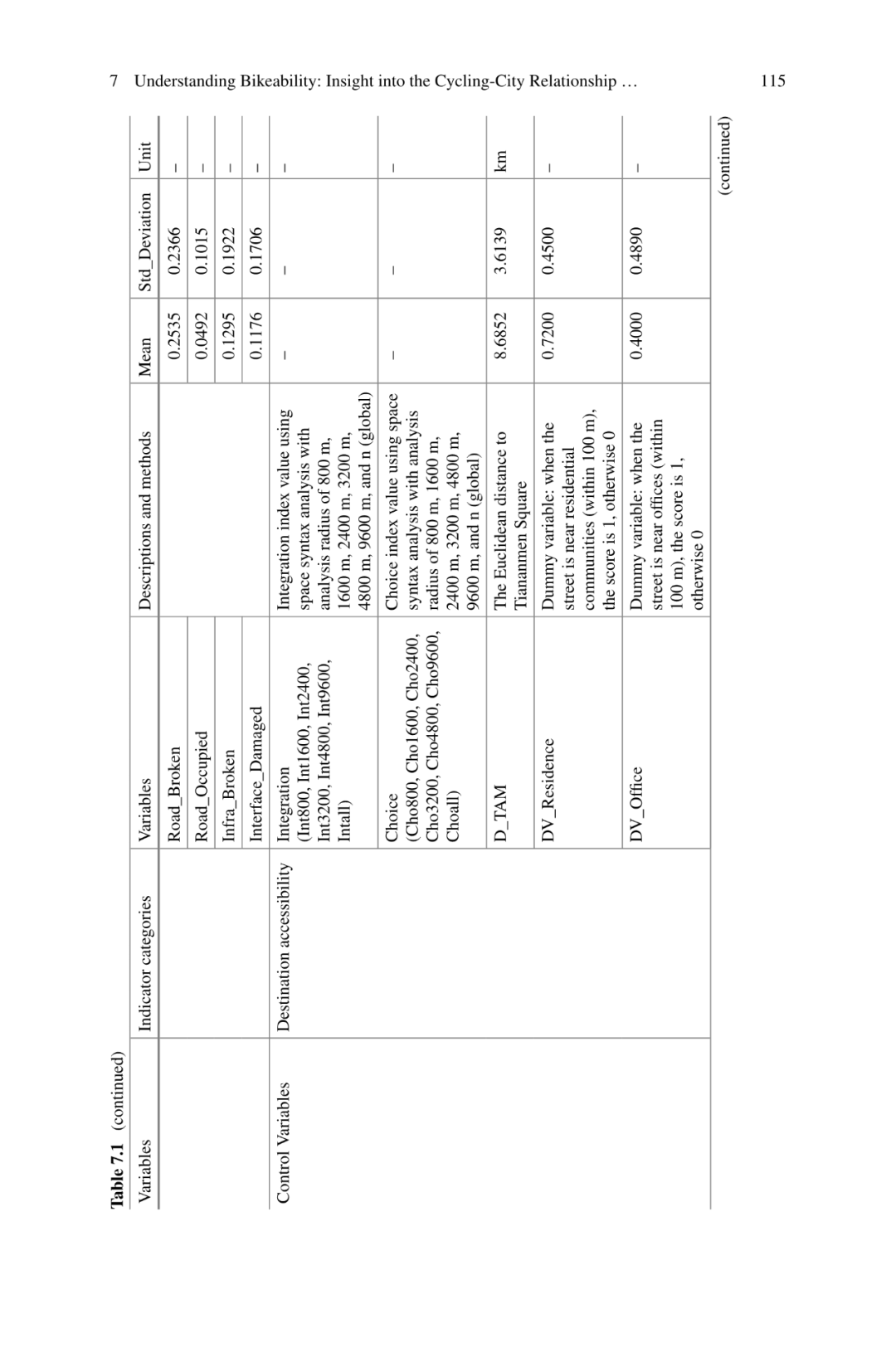
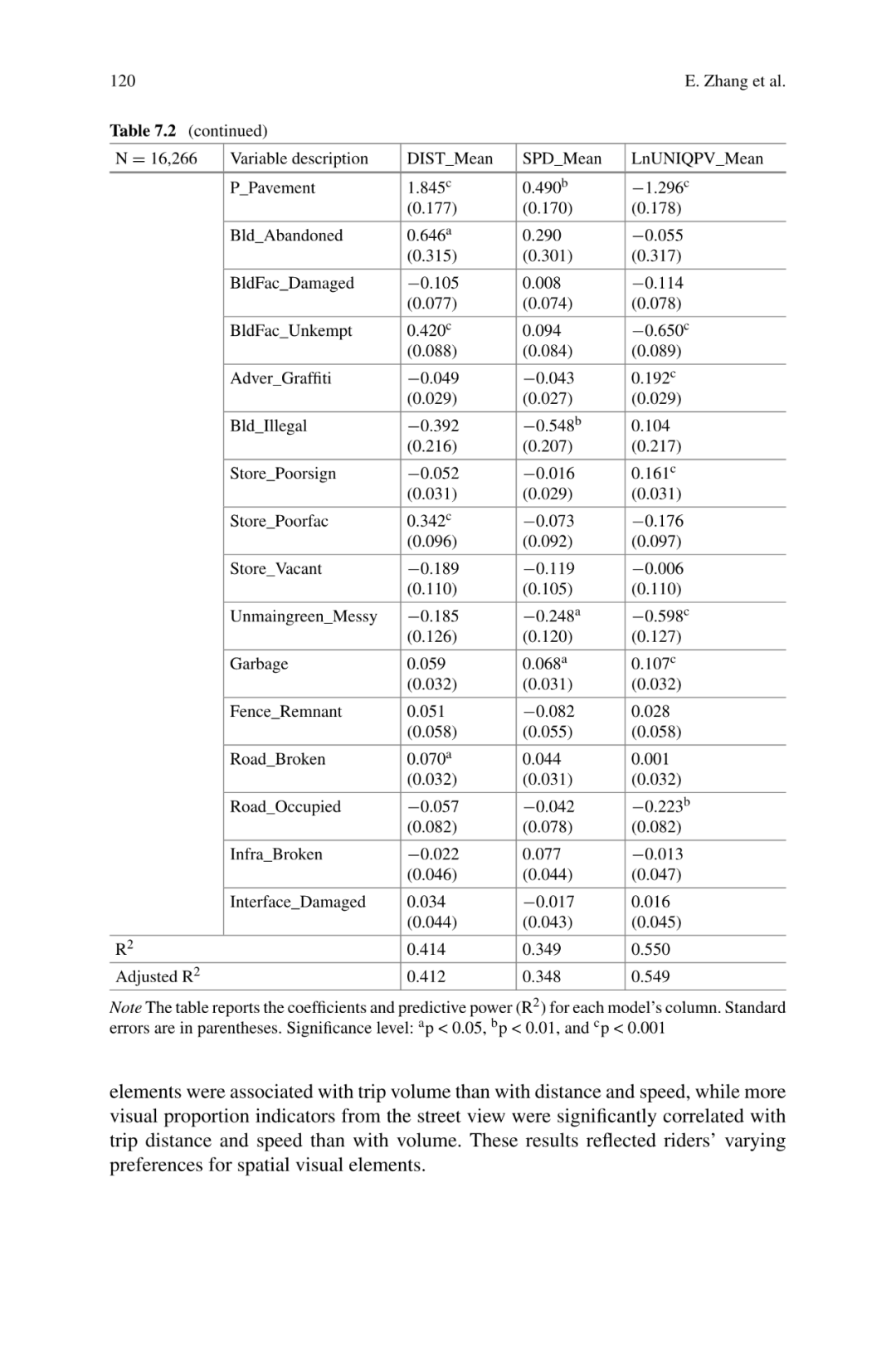
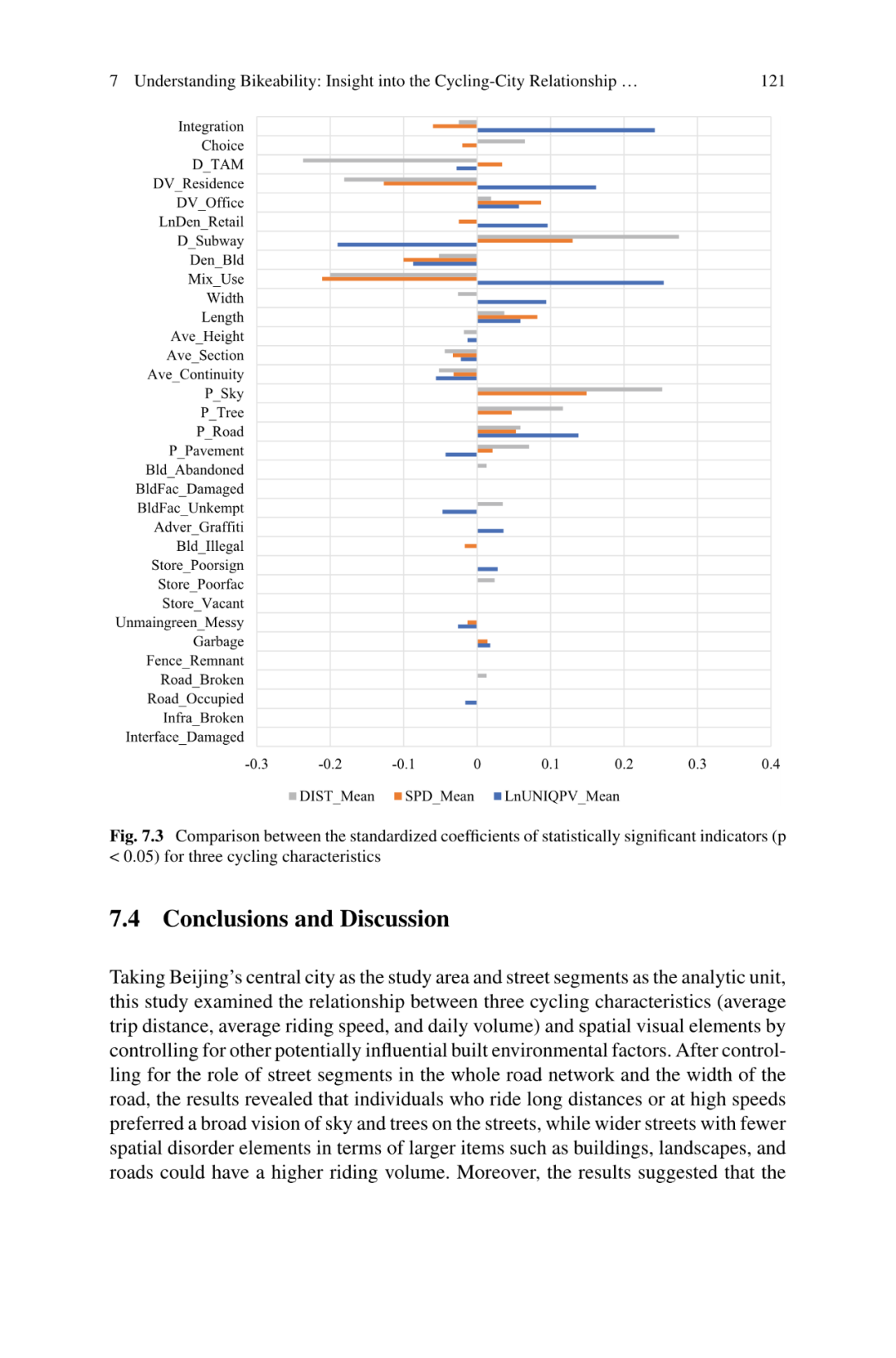

研究全文以及更多相关的研究工作详见BCL的【bike-landscapes】单元链接:
https://www.beijingcitylab.com/projects-1/41-bike-landscapes/
(复制至浏览器搜索或点击文末“阅读原文”查看)
BCL北京城市实验室“骑行景观” (bike-landscapes)项目还包括《用海量摩拜单车记录了解中国共享交通》、《摩拜单车骑行指数》等研究内容,欢迎探索。
论文《Understanding the Shared Transportation of China with Massive Mobike Records》(用海量摩拜单车记录了解中国共享交通),发表在BUILT ENVIRONMENT 期刊,欢迎大家学习与交流。
Abstract: Dockless bike share has been booming globally and in China. This paper aims to study what the mobile bike share data can help in terms of understanding our cities from the bikers’ perspective. The hypothesis is that mobile bike data would reveal a universal pattern in the bikeability distribution among Chinese cities. The research method is to construct a bikeability rating system, Mobike Riding Index (MRI), which measures bikeability in terms of usage frequency and the built environment. The first component of this paper is to investigate what Mobike ridership data entails and what relevant built environment data can be considered. The study of Mobike data found that people tend to ride shared bikes at a speed close to 10km/h, a length of 2km, and a frequency of three times a day. The implications to urban planners are addressed in the discussion. Following that is to establish the MRI framework and calculate its scores. After calculating the MRI, we studied the nationwide distribution of MRI in different analytical levels and discuss the noticeable patterns. Results show that at the street level, the weekday MRI distribution is analogous to that of the weekend’s with an average score of 49.8; at the township level, highly scored townships are those close to the city center; at the city level, the MRI distributes unevenly, with high MRI cities staying along the southern coastline or the middle inland area, such as Shenzhen, Chengdu, and Wuhan. This study is the first to incorporate mobile bike share data into bikeability measurement in China, and the largest in scale with 202 cities included, setting the ground for further research in this subject.
骑行是城市交通的模式之一,提高城市骑行友好性,可以带来诸多益处,如缓解交通压力,提高国民身体素质等。摩拜单车(Mobike)也致力于用摩拜数据更好的了解街道的骑行体验,提升绿色出行率。清华大学建筑学院龙瀛团队用2017年9月中一周的全国摩拜数据和相关街道信息计算并发布了摩拜骑行指数,Mobike Ridinglndex (MRI)。
请搜索微信号“Beijingcitylab”关注。
Email:BeijingCityLab@gmail.com
Emaillist: BCL@freelist.org
新浪微博:北京城市实验室BCL
微信号:beijingcitylab
网址: http://www.beijingcitylab.com
责任编辑:张恩嘉、张业成
原文始发于微信公众号(北京城市实验室BCL):论文推荐 | 理解城市可骑行性:利用北京大规模无桩共享单车数据洞察城市与骑行的关系