专刊推荐
本期为大家推介的是期刊《城市数据、科学与技术汇刊》(Transactions in Urban Data, Science, and Technology)2025年第1期(Volume 4 Issue 1)上收录的四篇文章。欢迎大家学习与交流!
Title: Revealing the city-scale spatial coopetitive relationships between themed and non-themed tourism attractions in three Chinese cities
Authors: Shaojing Wu and Zhixiang Fang*
Link: https://journals.sagepub.com/doi/full/10.1177/27541231251318347
Abstract:
The themes of cities and the types of attractions they offer significantly influence visitors’ decisions to explore specific destinations. However, systematic research on the competitive and cooperative (coopetition) relationships between thematic and non-thematic urban attractions remains scarce. This study analyzes the spatial patterns of coopetition relationships between thematic and non-thematic attractions in three Chinese tourist cities—Chengdu, Dalian, and Shenyang—during the 2020 National Day, based on pedestrian trajectory data provided by a service operator. By leveraging network analysis, geographic metrics, and a quantitative modeling approach, this paper introduces a novel framework that integrates network analysis with motif analysis to examine the interaction patterns between attractions. Specifically, the Fruchterman–Reingold algorithm is utilized to establish an analytical framework for thematic and non-thematic interactions among urban attractions. The results reveal both similarities and differences in the coopetition relationships of urban attractions. Across the cities studied, thematic attractions exhibit a strong tendency to cluster, while the competition among non-thematic attractions is more evenly distributed. The study is helpful to dynamically abstract the coopetition relationships and support the sustainable decision-making for urban tourism management.
Authors: Shuang Ma, Xuanyu Zhou, Wei Cai, Mo Chen, Shuangjin Li*
Link: https://journals.sagepub.com/doi/full/10.1177/27541231251314132
Abstract:
The post-pandemic era presents dual challenges for urban recovery: overcoming its impacts and shaping a new socio-economic pattern. Promoting urban recovery through non-pharmaceutical intervention is crucial. This study examines the impacts of built environment on urban socio-economic recovery in Metropolitan Nagoya in the post-pandemic period. Here we find that the geographical weighted random forest (GWRF) model has a better performance on urban recovery in central districts (kappa value is 67 ± 18%) compared to the peripheral areas in Nagoya, Japan. The balance of jobs and housing pre-pandemic emerges as crucial factor in urban recovery, especially in the low recovery level area. Moreover, accessibility to secondary roads notably promotes urban recovery in central area, while this factor also introduces the risk of lower recovery levels. By integrating nonlinear and spatial methodology, this study offers policymakers crucial insights into how different built environment can impact urban socio-economic recovery and environmental sustainability as the pandemic becoming frequently.
Title: Cross-platform complementarity: Assessing the data quality and availability of Google Street View and Baidu Street View
Authors: Lei Wang, Tianlin Zhang, Jie He, Martin Kada*
Link: https://journals.sagepub.com/doi/full/10.1177/27541231241311474
Abstract:
Google Street View (GSV) and Baidu Street View (BSV) cover most of the world’s cities. However, their combined use and research application still lack a unified standard. We selected Hong Kong Special Administrative Regions of China (SAR), a region where both types of street view image (SVI) are available, as the study area. In this study, we developed an SVI collection method based on a search-oriented approach. Using an SQLite database to manage over 700,000 SVIs, we conducted comparative experiments. Deep learning methods were employed to process the data and extract visual elements from both types of SVI. We performed a comprehensive comparison of the data quality and usability of the two SVIs in terms of temporal coverage, data acquisition efficiency, acquisition repeatability, and visual element similarity. The findings indicate that BSV outperforms GSV in terms of acquisition efficiency and repeatability, while GSV shows better temporal coverage. The results also confirmed high correlations between GSV and BSV in visual elements related to buildings, greenery, and sky, with correlation coefficients (R) of 0.781, 0.664, and 0.653, respectively. The comparative framework proposed in this study provides theoretical support for geoinformation researchers utilizing SVI data for global-scale studies.
Title: CGNet-PIE: A causal generative approach for urban planning intervention effect estimation
Authors: Zhou Fang*, Qianmu Zheng, Shuwen Zheng, Liang Zhao*
Link: https://journals.sagepub.com/doi/full/10.1177/27541231251318348
Abstract:
A critical challenge for urban planners lies in estimating the potential impacts of planning interventions before their implementation. Current approaches to urban planning intervention effect estimation encounter notable constraints. Association modelling approaches, while effective at capturing complex relationships between interventions and outcomes in observed cases, fail to estimate causal effects, making them unreliable for counterfactual predictions. Operational modelling approaches frequently set up structural causal models to transform interventions into outcomes based on subjective assumptions about simplified causal relationships. These approaches, while achieving a clear representation of causality, compromise the ability to accurately model the dynamic and intricate nature of cities and human behaviour. This paper introduces the Causal Generative Network for Planning Intervention Effect estimation (CGNet-PIE), a lightweight yet robust approach that directly estimates causal effects from observed data without the need for fully articulated causal interaction models. By integrating generative deep learning with the Rubin Causal Model, CGNet-PIE effectively captures non-linear relationships and enhances causal inference for reliable and generalizable counterfactual predictions. Experiments on synthetic, semi-synthetic, and real-world datasets demonstrate CGNet-PIE’s superior accuracy and generalization compared to baseline models. CGNet-PIE offers a powerful tool for planners to improve decision-making for informed policymaking and optimal resource allocation.
【期刊主页链接】:
https://us.sagepub.com/en-us/nam/transactions-in-urban-data-science-and-technology/journal203731

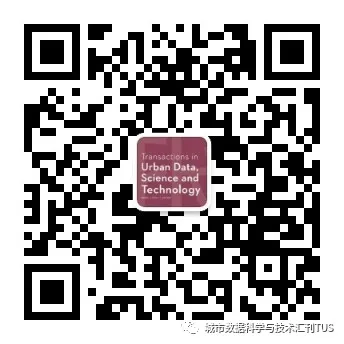

更多内容,请点击微信下方菜单即可查询。
请搜索微信号“Beijingcitylab”关注。
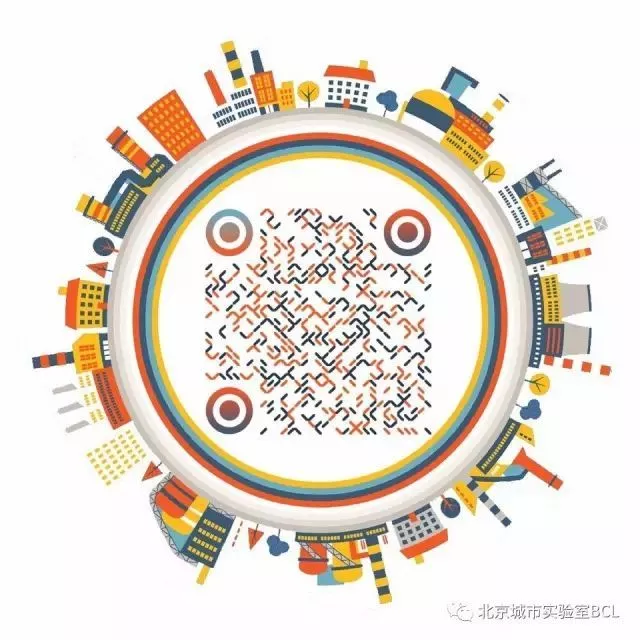
Email:BeijingCityLab@gmail.com
Emaillist: BCL@freelist.org
新浪微博:北京城市实验室BCL
微信号:beijingcitylab
网址: http://www.beijingcitylab.com
原文始发于微信公众号(北京城市实验室BCL):期刊系列推荐 | 《城市数据、科学与技术汇刊》2025第1期