著名的张帆师弟近些年专注于城市问题的研究,多项工作对我都很有启发,所以他主持的这期专刊征稿也是第一时间看到。当时王磊和自己都是刚刚决定面向各种不确定之下来用这一个博士生配额,本想也许王磊可以把他硕士的工作再发展一下可以去投(不过最后我看了论文终稿还是觉得重新做一个是好主意)。过程就不复述了,流程其实不算长(只不过我们近期发的其它都太快了)。发个关联行业顶刊是个好兆头、尤其在大家越看新提出来的破若干维用顶刊列表都赶脚是明晃晃是鄙视自己一亩三分地学科的这几天,地学和遥感俩字毕竟是常年亲切的东西,另外博士生一发入魂可以毕业。不过文发出来攻防立刻转换,自己要承认research question还是老大难问题。街景的研究方向上,我自己应当是又如当年建成环境行为的领域一模一样,于如此的卷王话题下迅速开头迅速攀升然后迅速做个逃兵,还要请非常厉害的小同事边老师顶上一下帮着琢磨新突破。
中日韩之中国篇。需要说明的是和已经发表的韩国篇,在研究中的日本篇研究逻辑之间没有必然的联系。不过作为我研究三部曲中的三个选地,同属于亚洲影响力最大的三个国家,未来希望找到一个有趣的切入点讨论在这一相似文化背景前提下城市感知特征的异同。如果我的野心是Nature子刊,这一范围至少还要扩大至世界多个洲的城市。回到研究本身的主题,我们发现诸多研究对某一类/几类感知分布进行讨论,但每类感知仅能反映人类对城市的片面感受,如果将多类高感知进行叠加并使用空间句法进行高可达性的限定,是否能产生一个足够有趣的结果并进行讨论?便是这篇文章的开端。
这是我博士入学以来正经做的第一个工作,发表的第二篇SCI论文。经历了两次大修一次小修,备份了67个中英文历史修改版本,绘制配图源文件258个,产生10G源数据。修改过程中Abstract反复推敲、Methodology冗余、Result描述格式错误、Discussion讨论过少。第一次翻译文章、第一次写CoverLetter、第一次写Review回复。为了学习这些现在看来的常识,我阅读了上百篇相关方向文章,无数次地修改代码,请教身边的老师同学。从2021/02/10收到导师通知专刊征稿,到2021/12/29压着deadline投稿,再到2022/06/28文章接受。真正的一年磨一剑。这一过程比开始时我想象中要困难得多,但我还是没有学会“放弃”两个字怎么写,这也都成为了我在学术游戏中升级所必须的经验值。
感谢我的导师何捷老师悉心指导,从开始不成样的初稿耐心指导修改至最终接收。感谢合作者韩鑫博士和我共同完成了研究。感谢MIT Senseable City Lab张帆老师对文章的责编,并给出的专业修改建议。感谢两位匿名审稿人提出的审稿意见。
实验室于2022年6月在地学、自然地理、影像与遥感等领域的顶级期刊《ISPRS Journal of Photogrammetry and Remote Sensing》发表学术论文《Measuring residents’ perceptions of city streets to inform better street planning through deep learning and space syntax》,收录于该刊“Street-level Imagery Analytics and Applications”专刊中。该论文由何捷老师指导,实验室2021级博士研究生王磊同学与庆北大学(韩国)合作者共同完成。
本期推送对该文进行简要译介以飨读者。点击文末“阅读原文”可跳转至《ISPRS Journal of Photogrammetry and Remote Sensing》网络发表界面,欢迎交流和指正。
通过深度学习和空间句法测量城市居民感知:为更好的街道规划提供信息
Measuring residents’ perceptions of city streets to inform better street planning through deep learning and space syntax
Wang L, Han X, He J and Jung T (2022) Measuring residents’ perceptions of city streets to inform better street planning through deep learning and space syntax. Front. ISPRS Journal of Photogrammetry and Remote Sensing 190, 215–230.
https://doi.org/10.1016/j.isprsjprs.2022.06.011
关注城市数据派微信号,在对话框中输入:1023 ,即可获得论文全文下载地址。
良好的街道空间品质对于促进城市发展起到了重要的作用。街道空间指包括植物、道路、建筑等街道要素构成的公共空间。人是街道空间的使用主体,如何以人的感知视角去量化街道空间品质,探索街道空间品质与街道构成元素之间的联系一直是各个领域所致力研究的课题。大数据和计算机学科的发展为定量评估街道感知提供了全新的技术手段,而空间句法可以为精细化街道空间感知研究提供理论补充。本文探索了一种依托街道空间感知来大面积评测街道空间品质的新方法。以杭州市滨江区为研究对象来验证我们的方法。利用该区域的街景图片,构建深度学习评分模型。从美丽、富裕、安全、活泼、压抑、无聊六个维度进行感知打分。将六个维度感知进一步分为积极感知与消极感知。积极感知得分最高的前20%街景作为高品质街道空间,消极感知得分最高的前20%街景作为低品质街道空间。最后叠加高可达性识别了街道中居民穿行概率最高且品质高(低)的街道空间。而且,我们利用多元线性回归解释了街道空间品质与街道构成要素之间的联系。研究结果表明,在街道高可达性区域中,城市中积极感知空间和消极感知空间均呈现聚集分布的趋势。积极感知与植物、道路要素呈正相关关系,与墙、地面、水体、围栏要素呈负相关关系。消极感知与墙、建筑要素呈正相关关系。这一结果为揭示城市空间感知规律提供了基础信息。空间句法运用在深度学习中可以为更精细化地城市规划研究提供方法补充,为以后以人的感知视角进行城市规划提供参考。
为了促进未来的研究和这一创新的传播,该研究的源数据和代码可以在https://github.com/LandscapeWL/SHAPClab_UrbanPerception_StreetAccessibility找到。
The quality of street space plays an important role in promoting urban development. Street space refers to public spaces consisting of street elements such as plant, roads and buildings. Humans are the main users of street space. How to quantify the human perception of the quality of street space and how to explore the connection between the quality of street space and street composition elements have been major topics of research in various fields. The development of big data and computing has offered new technical tools for the quantitative assessment of street perception, while space syntax can provide a theoretical complement to fine-grained spatial perception studies for streets. Our research introduces a new method to evaluate the quality of street space on a large scale based on street space perceptions. The Binjiang district of Hangzhou city of Zhejiang Province of China was used as the study area to validate our method. A deep learning scoring model was constructed using street images of the area. The perception of the street scenes was scored on six dimensions: beautiful, wealthy, safety, lively, depressing, and boring. The six perceptual dimensions were further divided into positive and negative perceptions. The top 20% of street views with the highest positive perception scores were considered high-quality street spaces, and the top 20% of street views with the most negative perception scores were considered low-quality street spaces. Finally, an overlay of those streets with the highest accessibility identified street spaces with the highest probability of travel and the highest or lowest quality for residents. These streets are of high priority in the subsequent urban plan. We used multiple linear regression to explain the association between the spatial quality of the streets and their constituent elements. The results showed that positive perceptions were positively correlated with the presence of plants and roads and negatively correlated with walls, the ground, water, and fences. Negative perceptions were positively correlated with walls and buildings. The present study provides fundamental information on the patterns of urban spatial perception. The use of space syntax in deep learning can provide methodological support for more refined urban planning research and provide a reference for future urban planning that embraces a human perspective.
To facilitate future research and the dissemination of this innovation, source data and code of the research can be found in https://github.com/LandscapeWL/SHAPClab_UrbanPerception_StreetAccessibility.
街景图片 语义分割 空间句法 街道质量 深度学习 机器学习 城市感知
Street view, Semantic segmentation, Space syntax, Street quality, Deep learning, Machine learning, Urban perception
Fig 1. Research framework for the present study.
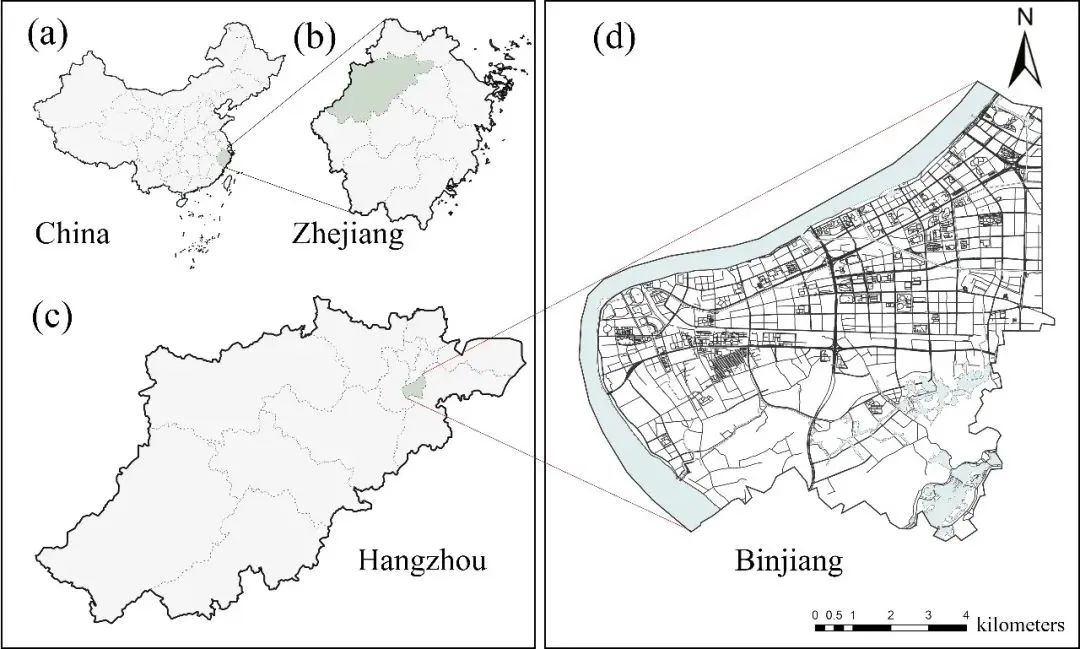
Fig 2. Overview of the study area for the present study:
(a) location of Zhejiang Province in southern China,
(b) location of Hangzhou within Zhejiang Province,
(c) administrative districts of Hangzhou, highlighting Binjiang, and (d) the road network in Binjiang.
图2 本研究的研究区域概述:(a)中国南部浙江省(b)浙江省杭州市(c)杭州市滨江行政区(d)滨江区道路网络
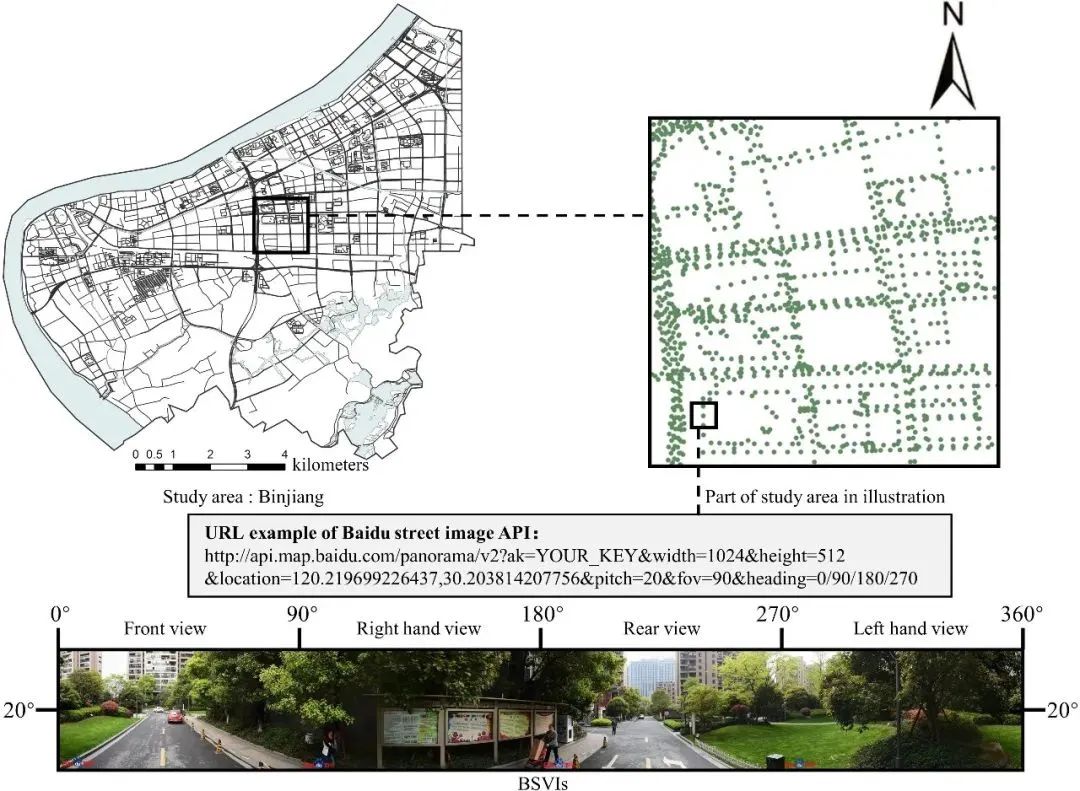
Fig 3. Example of the BSVIs collection process.
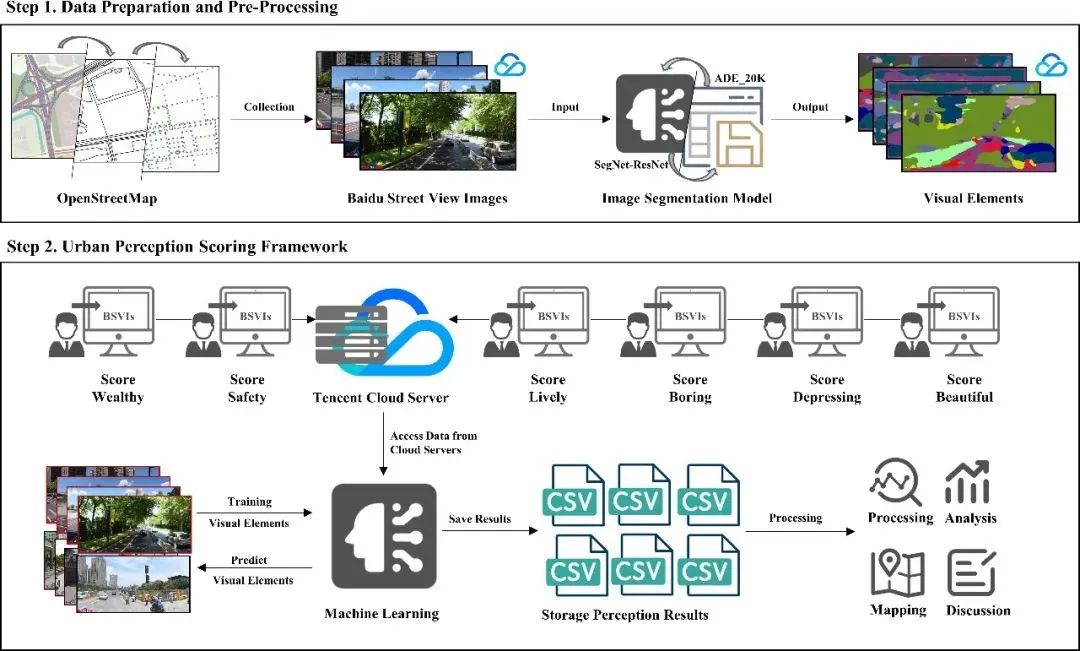
Fig 4. Overview of the spatial perception scoring process for urban streets based on a machine learning.
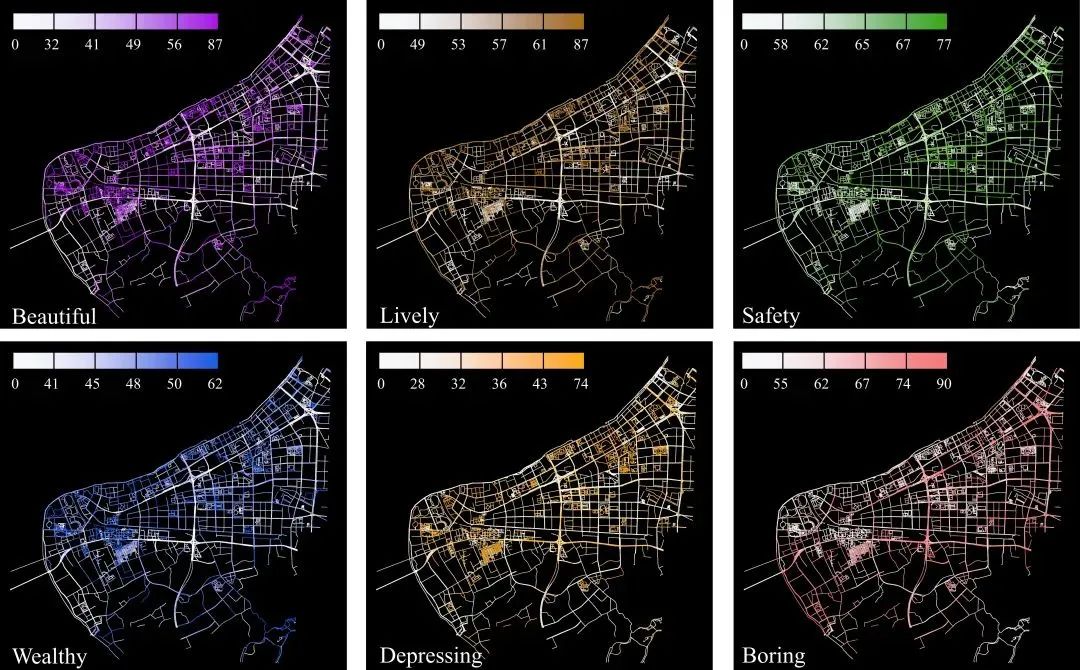
Fig 5. Analysis of the six dimensions of emotional perception in the Binjiang district.
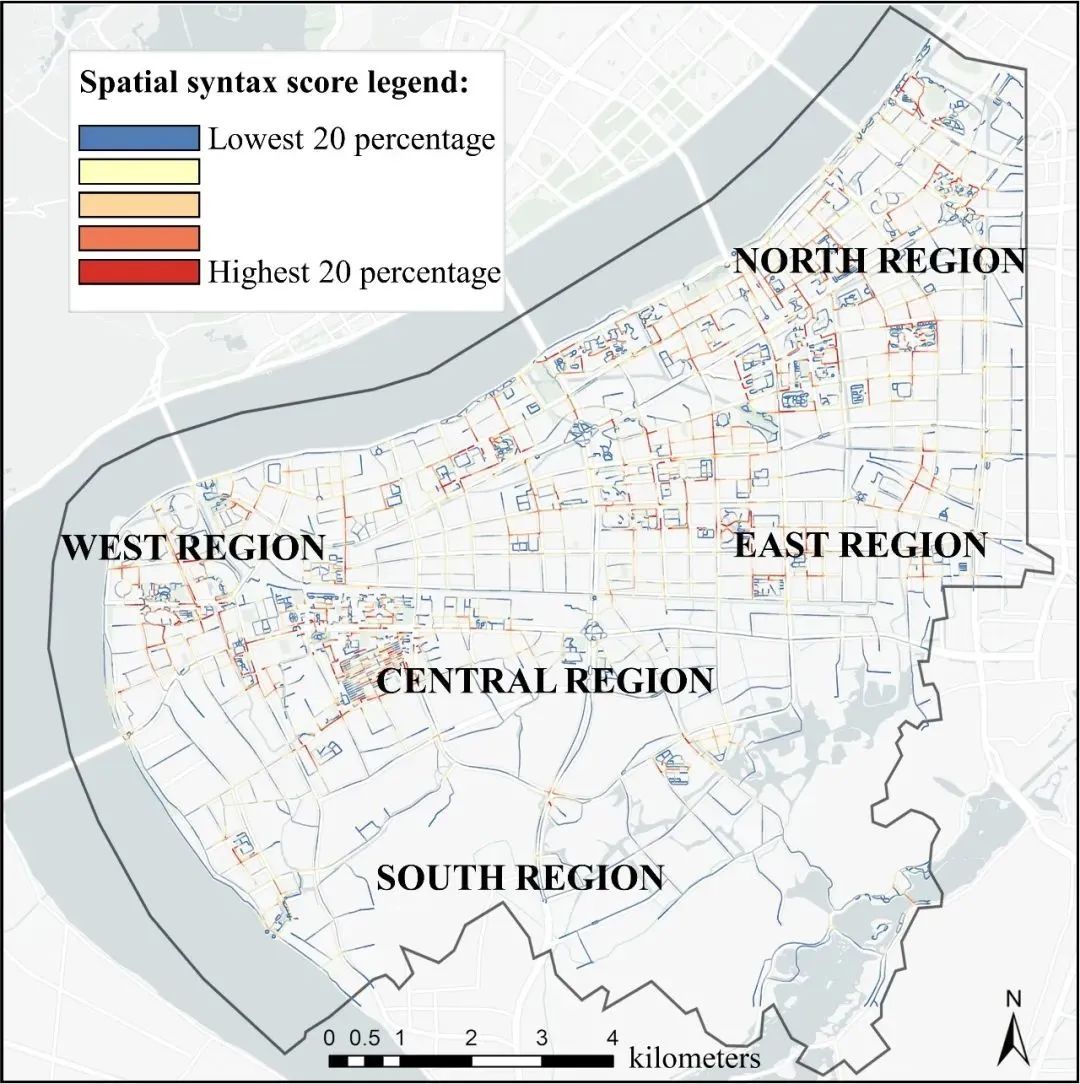
Fig 6. Accessibility of the streets within a walking distance of 500 m for urban residents in the Binjiang district.
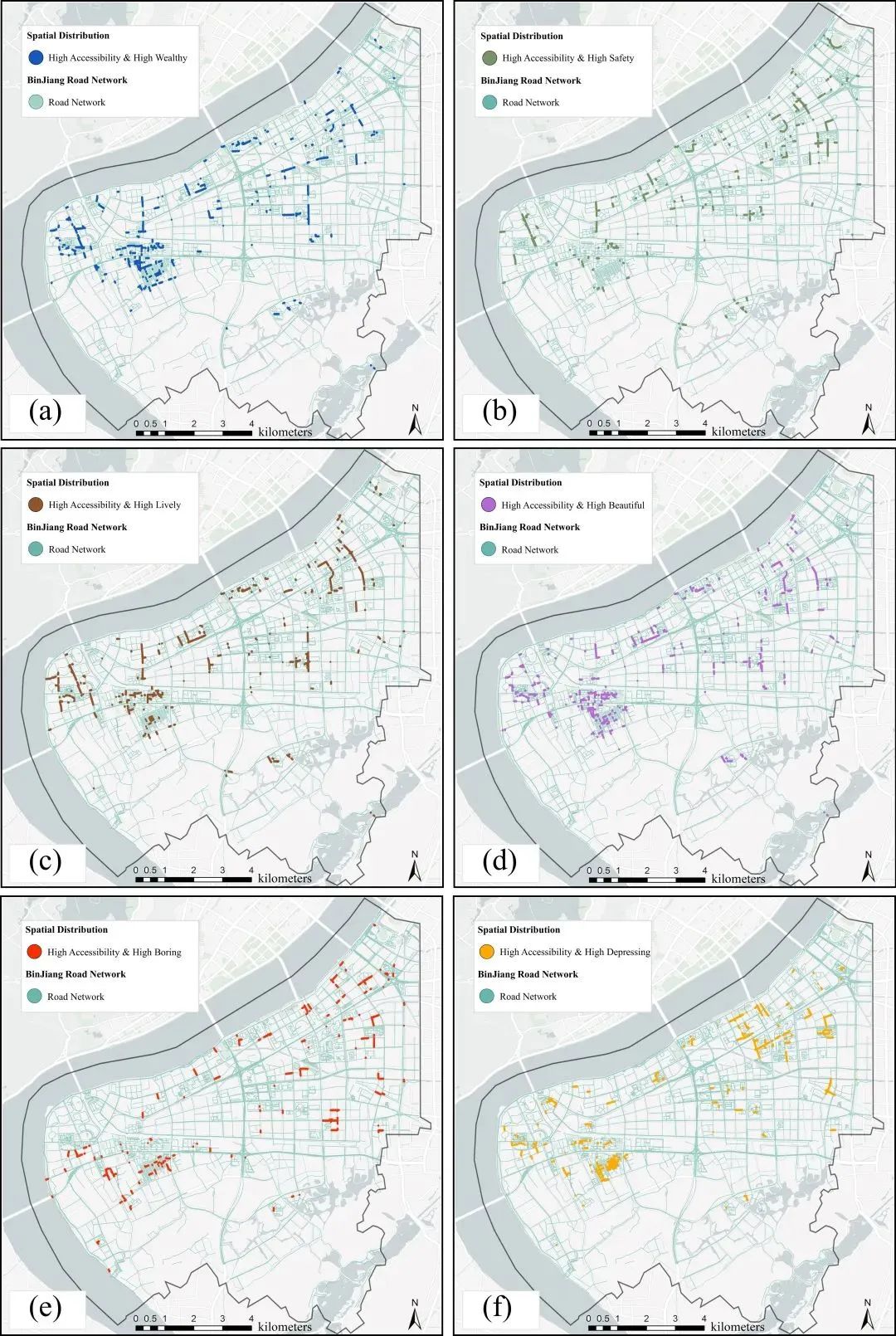
Fig 7. Overlay of the top 20% streets with high accessibility and the top 20% streets with high spatial perception scores for (a) wealthy, (b) safe, (c) lively, (d) beautiful, (e) boring, and (f) depressing in the Binjiang district.
图7 高可达性的前 20% 街道和高空间感知得分前 20% 街道叠加图:(a) 富裕 (b) 安全 (c) 活泼 (d) 美丽 (e) 无聊 (f) ) 压抑

Fig 8. Distribution map of positive and negative perceptions of street views in the Binjiang district.

Fig 9. Analysis of the visual elements associated with the high perception scores in street views for six perceptual dimensions.
图9 六个感知维度的街景中与高感知得分相关的视觉元素分析
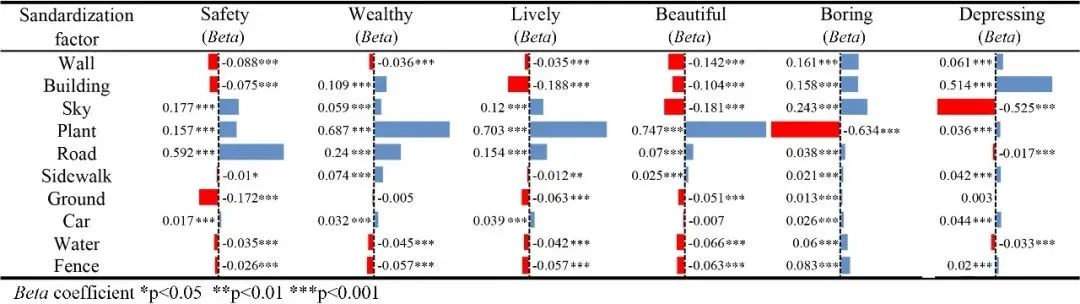
Fig 10. Results of the multivariate linear regression analysis for the visual elements and perception scores.
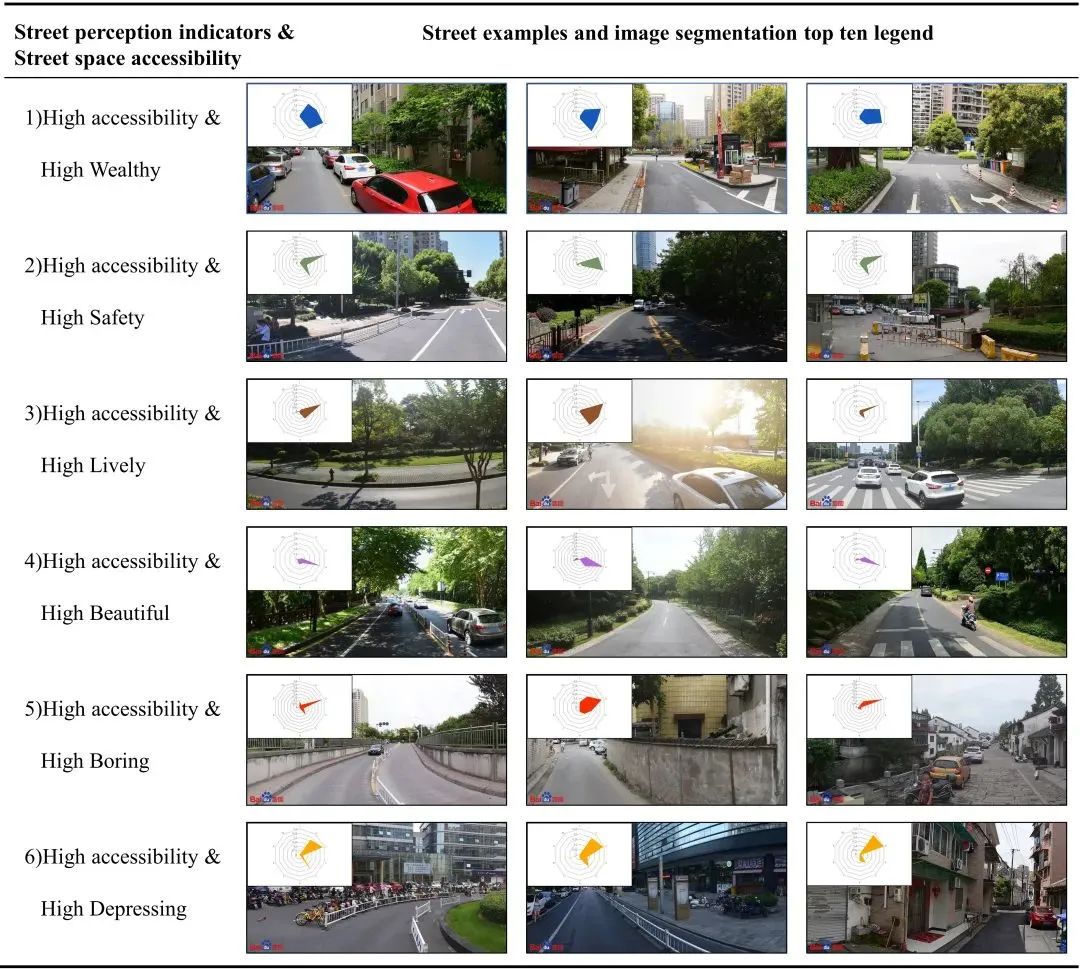
Fig 11. Representative street view images for different perceptual dimensions. The decagon in the top left represents each of the ten visual elements from image segmentation: Clockwise from the vertex. (a) walls, (b) buildings, (c) the sky, (d) plant, (e) roads, (f) sidewalks, (g) the ground, (h) cars, (i) water, and (g) fences.
图11 不同感知维度的代表性街景图像。左上角的十边形代表来自图像分割的十个视觉要素:顶点顺时针方向起 (a)墙壁(b)建筑(c)天空(d)植物(e)道路(f)人行道(g)地面(h)汽车(i)水体(g)围栏
最近有朋友问我们:为什么没有及时看到推文?因为微信改了推送规则,没有点“赞”或“在看”,没有把我们“星标”,都有可能出现这种状况。
加“星标”,不迷路!看完文章顺手点点“赞”或“在看”,就可以准时与我们见面了~
原文始发于微信公众号(城市数据派):通过深度学习和空间句法测量城市居民感知:为更好的街道规划提供信息丨城市数据派